The Potential of Data Analytics
In the digital age, data is frequently likened to oil, a valuable resource that, when refined and analyzed, can yield powerful insights. Data analytics involves the meticulous examination of large datasets to uncover hidden patterns, correlations, and trends, thereby enabling businesses to make data driven decisions.
Descriptive analytics involves the summarization of historical data to comprehend past events, utilizing techniques such as data aggregation and data mining. Diagnostic analytics examines the data more thoroughly to ascertain the reasons behind specific occurrences, often employing statistical analysis to identify root causes. Predictive analytics leverages statistical models and machine learning techniques to forecast future trends based on historical data. Prescriptive analytics extends this approach by recommending actions based on these predictions, thereby optimizing decision making processes.
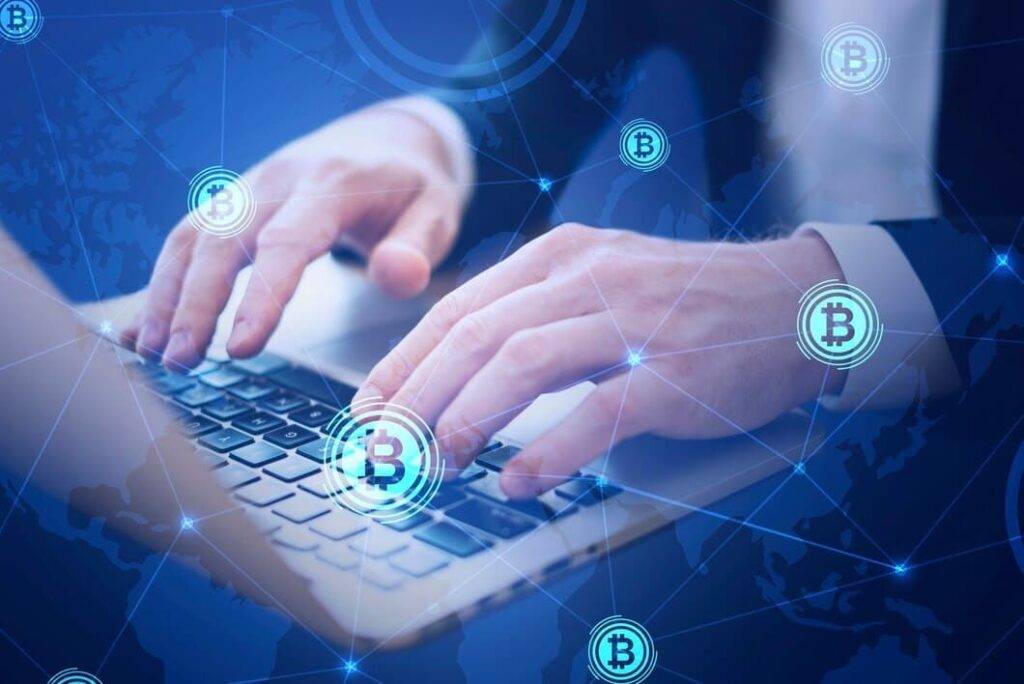
Data mining involves extracting patterns and knowledge from large sets of data, often utilizing machine learning, statistics, and database systems. Statistical analysis employs statistical methods to analyze and interpret data, aiding in understanding relationships and trends. Machine learning utilizes algorithms to learn from data, enabling predictions or decisions without explicit programming. Data visualization involves creating visual representations of data, making complex information more accessible and understandable.
Python and R are popular for data science because they have many tools and are easy to use. Programs like Tableau, Power BI, and D3.js help create interactive visuals. SQL is used for regular databases, while MongoDB and Cassandra handle large amounts of data. Hadoop and Spark are used for big data because they can process and analyze large datasets across many computers. TensorFlow, Scikit-Learn, and PyTorch are used to build and use machine learning models. These tools help people in different industries make better decisions with data.
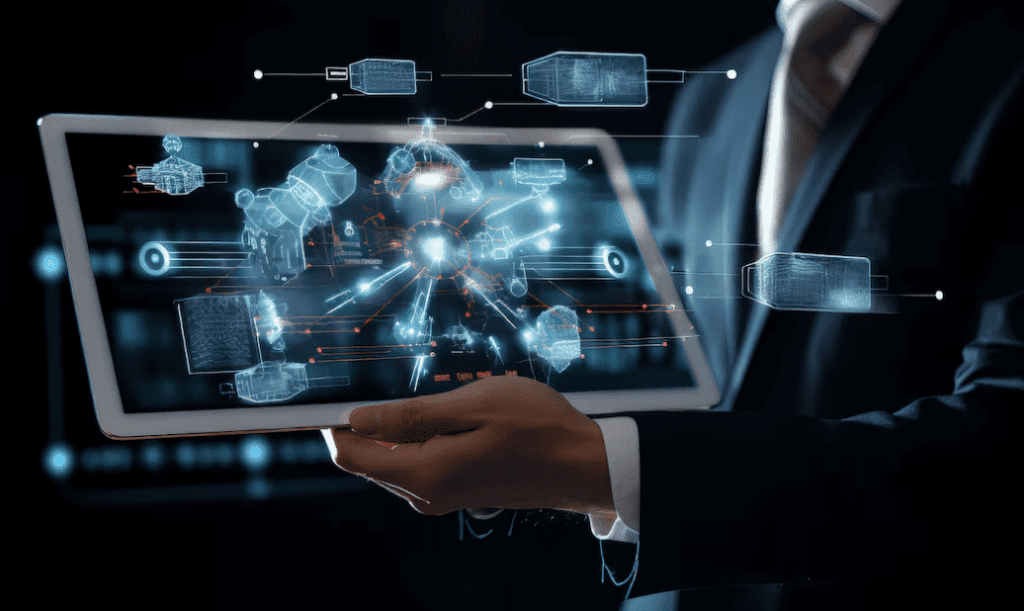
Predictive analytics is incredibly useful across different industries. In healthcare, it helps by predicting health issues ahead of time and making treatment plans better, which leads to improved patient outcomes. In finance, it uses real-time data to manage risks and catch fraud more effectively. Retailers use it to understand what customers want and manage their stock better. And in manufacturing, it helps prevent equipment breakdowns by analyzing data to find problems before they cause issues.
Ensuring data is accurate, complete, and consistent is crucial for reliable analytics. Protecting sensitive information and following rules like GDPR and CCPA is a big concern. Bringing together data from different sources and types can be complicated and needs strong solutions. Handling large amounts of data efficiently requires scalable infrastructure and advanced technology. These factors data accuracy, privacy, integration, and scalability are essential for organizations to use data effectively for making smart decisions. By focusing on these areas, organizations can ensure their data-driven operations are efficient, secure, and compliant with regulations, maximizing their strategic value.
Concluding this article by stating, Data analytics is crucial for businesses to use data effectively for making smart decisions and driving innovation. By understanding how to analyze data, using the right tools, and solving challenges that come up, companies can discover new opportunities and stay ahead of the competition. As technology keeps advancing, data analytics continues to grow in importance, becoming a key part of modern business strategies.
-written by Aathil Ahamed A